Is there a magic wand for Analytics
The latest buzzwords around Analytics industry are ‘AI’ and ‘Machine Learning’, but is this some kind of a magic wand for predicting future like the Oracle from Ancient Greece? Well, some of you may think that there is a bit of similarity in the approach but it differs in the way data is collected. Let’s put it this way, if the right questions are not asked, then we may not have the right answer available to us. For eg. if the warriors of Greece asked Oracle “will i win the war ?” Oracle would certainly not tell them about “how you need to fight or strategise”
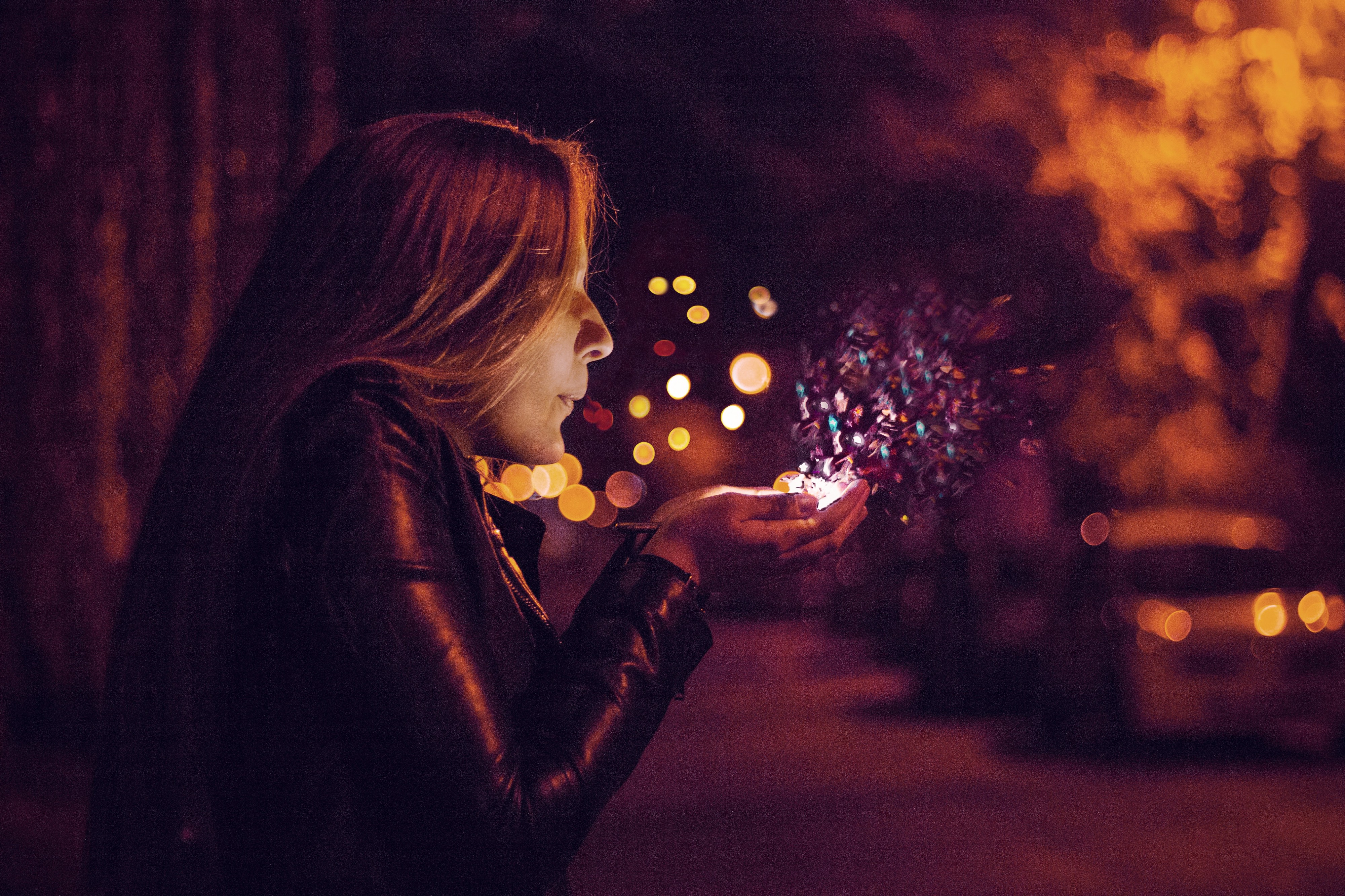
The world of Big Data preachers, which would also include me 🙂 , have always talked about delivering value out of the available primary and secondary data but what if the available data around a customer is not that detailed enough? Will it still deliver the right value??
I came across an ethnographer Tricia Wang, in fact a popular one, who says that adding behavioural and cultural phenomenon to data, thereby adding the “thick value” to it, would give data analytics a different dimension than the existing ones. Analysing just the raw transaction data available may not suffice !
One of her researches is a fascinating study on Nokia and how they could not foresee the explosion in popularity of smart phones, even when they had big research analysts studying their data and sales patterns. This ultimately resulted in the dramatic downfall of Nokia against its Chinese counterparts. Why they could not predict was not because of faulty algorithms, but because they never asked the right questions. If you don’t ask about the features desirable in a smart phone; people will not turn their attention towards it as they have never even seen it. The same goes for a Car survey wherein if you don’t ask people about their views on battery/electric cars, they might not willingly express their preference for them. So big data today definitely needs to be thicker to achieve better decision making results. Asking the right question matters the most, and ethnographers have an important role to play.
“Asking the wrong questions and lack of business outcome has led to the failure of 70–80% of Big Data projects.”
Most companies want to establish a Big Data setup today without a strong business objective or outcome in mind. They would rather place multiple outcomes or the clichéd big data use cases in front of them to measure the ROI on their big data strategy, which makes them vulnerable to failure. The best way to tackle the problem is to think of the desired outcome and then back track it to a single use case. This use case could then be reverse engineered or broken into multiple milestones.
For e.g. to achieve a use case of ‘Personalised Campaigning’, you first need to reach a milestone of Customer or Analyse 360, for which you need to have a mechanism through which you are able to collect customer data, sort it, segment it and finally profile it based on buying or social patterns. But the benefits will succumb if data is not available real time or near real time plus if its not “Varied Enough”. Churning data real time would effectively scale analytics to a level of campaigning which would matter to the consumer. So our main business objective here would be ‘increasing sales revenue’, the translated use case is ‘Personalised Campaigning’, foundation milestone is ‘Customer 360’ and the sub milestones are ‘Segmentation’, ‘Sorting’ and ‘Profiling’ @ a near Real Time level. If our planning sheet goes like that, I don’t see a reason for the project failing. The flaw always lies in the questions we ask and how quick we fulfil the answers because we are living in a world where conditions keep changing and hence the behaviour. The idea is to visualize a data point as soon as it is born. Thanks to social media platforms like Facebook which present us with certain amount of ethnographic information about a person ,which, if combined with the primary data available like age, gender, marital status, religion, etc could point to a group behaviour. This behavioural data can then be combined with the transactional customer data to generate a holistic customer view along with a customer lifecycle. This data if used by AI engines could do wonders in terms of recommendation, in turn increasing sales revenue.
Going back to my initial thought of “Magic Wand for Analytics”, the idea is to have a Single Source of Truth which performs real time extraction and transformations to generate a customer lifecycle or 360 view, giving analysts a cutting edge platform to focus on algorithms which can then provide faster recommendations for marketing teams to react in time. According to the new market research report “Streaming Analytics Market — Global Forecast to 2021”, published by MarketsandMarkets™, streaming analytics market is estimated to grow from USD 3.08 Billion in 2016 to USD 13.70 Billion by 2021, at a Compound Annual Growth Rate (CAGR) of 34.8%. For this industry to succeed you would definitely need a Single Source of Truth !